- About-News
- Team
- Collaborations-Grants
- Publications
- Student Theses/Projects
- Vacancies
- Teaching
- Tools
- Contact
Summer 2024 Internship Program for Students from India
The application deadline is September 30, 2023. Please send your application (CV and grade sheet) to cpd@tu-dresden.de. The applicants should also specify the two intended projects from the list.
Master Thesis/Project, SHW/WHK, Internship positions
We are always looking for highly motivated students to join our team on topics of Approximate Computing, Machine Learning, Design Automation for Emerging Technologies, Reliability and Fault-Tolerance, etc. Please see the project herein and do not hesitate to contact us.
Compared to ASIC and FPGA, CGRAs is a more viable hardware platform for implementing applications in IoT edge devices, due to their promising trade-off in performance and energy-efficiency. In our recent papers (see one here), we have designed an approximate CGRA which can execute various applications from biomedical to image/video processing. For the follow-up journal paper, we want to extend the mapping to support for 5G applications. So we are looking for a 3-6 month student assistant, who will be a co-author in this hot topic.
Tasks:
- Generate the Data Flow Graph (DFG) of applications using e.g., LLVM compiler
- Mapping applications' DFG using Morpher/OpenCGRA mapper
Requirements:
- Verilog/VHDL
- Experience with ASIC mapping tools (Morpher, OpenCGRA, CGRA-ME, etc)
- DFG generation using LLVM
- Installation of open-source (github) tools on Ubuntu
Contact information for more details
The run-time reconfigurability and high parallelism offered by FPGAs make them an attractive choice for implementing hardware accelerators for ML algorithms. In the quest for designing efficient FPGA-based hardware accelerators for ML algorithms, the inherent error-resilience of ML algorithms can be exploited to implement approximate hardware accelerators to trade the output accuracy with better overall performance. As multiplication and addition are the two main arithmetic operations in ML algorithms, most state-of-the-art approximate accelerators have considered approximate architectures for these operations. However, these works have mainly considered the exploration and selection of approximate operators from an existing set of operators. To this end, this project focuses on designing a reinforcement learning (RL)-based framework for synthesizing and implementing novel approximate operators. RL is a type of machine learning where an agent learns to perform actions in an environment to maximize a reward signal. RL-based techniques would help achieve approximate operators with better accuracy-performance trade-offs in this project.
- Pre-requisites:
- Digital Design, FPGA-based accelerator design
- Python, TCL
- Some knowledge of ML algorithms
- Skills that will be acquired during project work:
- ML for EDA
- Multi-objective optimization of hardware accelerators.
- Technical writing for research publications.
- Related Publications:
- S. Ullah, S. S. Sahoo, and A. Kumar. "CoOAx: Correlation-aware Synthesis of FPGA-based Approximate Operators." Proceedings of the Great Lakes Symposium on VLSI 2023. 2023.
- S. Ullah, S. S. Sahoo, N. Ahmed, D. Chaudhury, and A. Kumar "AppAxO: Designing App lication-specific Approximate Operators for FPGA-based Embedded Systems." ACM Transactions on Embedded Computing Systems (TECS) 21.3 (2022): 1-31.
- S. Ullah, S. S. Sahoo, A. Kumar, "CLAppED: A Design Framework for Implementing Cross-Layer Approximation in FPGA-based Embedded Systems", In Proceeding: 2021 58th ACM/IEEE Design Automation Conference (DAC), pp. 1-6, Jul 2021.
- Contact: Salim Ullah
Various open-source tools, such as HLS4ML, FINN, and Tensil AI, enable fast FPGA implementation of machine learning algorithms. In the quest for designing efficient FPGA-based hardware accelerators for ML algorithms, the inherent error-resilience of ML algorithms can be exploited to implement approximate hardware accelerators to trade the output accuracy with better overall performance. However, the available hardware generators always generate implementations that employ accurate arithmetic operators. This short project focuses on extending these frameworks to consider approximate arithmetic operators for various operations in the generated RTL of the algorithm. In this regard, our chair offers extended libraries of FPGA-optimized approximate operators, which would be utilized in the project.
- Pre-requisites:
- Digital Design, FPGA-based accelerator design, High-level Synthesis
- Python, C++
- Skills that will be acquired during project work:
- Hardware design for ML
- System-level design
- Technical writing for research publications.
- Related Publications:
- S. Ullah, S. S. Sahoo, N. Ahmed, D. Chaudhury, and A. Kumar "AppAxO: Designing App lication-specific Approximate Operators for FPGA-based Embedded Systems." ACM Transactions on Embedded Computing Systems (TECS) 21.3 (2022): 1-31.
- Contact: Salim Ullah
Federated Learning and Distributed Inference are key enablers for real-time processing in 5G/6G era. To enable such compute-intensive workload at the edge, the structure of NNs should be optimized without compromising the final quality of results. In this context, Approximate Computing techniques have shown to provide highly beneficial solutions by exploiting the inherent error resiliency of ML applications. Considering such potentials, the main idea in this project is to apply various approximations, efficiently, to reduce the area/power/energy of NNs and boost their performance.
Pre-Requisites and helpful skills
- FPGA development and programming: Verilog/VHDL,
- High-Level-Synthesis:Vivado/Vitis HLS
- ML: C++/Python and Tensorflow/PyTorch to implement and modify the structure of NN models
Contact information for more details
As the classical digital computers approach the fundamental limit of scaling with Moore’s law, the search is on for alternative non-traditional hardware platforms and architectures. An entirely different computing philosophy is given by analog computing, which relies on a continuous physical quantity to model the computation. Several systems can support computing in the analog domain ranging from optical and electronic computers to molecular and fluid computers. The most prominent example is the electronic analog computers with resistors, inductors, capacitors and op-amps as basis components that can be used for analog operations, such as addition, subtraction and integration, forming the instruction set. Optical analog computers are an attractive paradigm as they offer energy-free linear operations at the speed of light. Such computers can perform complex calculations with available instructions but at the cost of accuracy and noise due to repeated processing of analog signals. In this context, Approximate Computing techniques can provide advantages by exploiting the inherent error resiliency of ML applications. The main idea within this project is to develop approximations and error correction techniques in the analog domain, particularly to enable new algorithms and applications for optical computers.
Pre-Requisites and Helpful Skills:
- Computer Architecture, preferably analog and mixed-signal design
- Programming languages (C++, Python)
- Knowledge about machine learning techniques
Skills that will be acquired during project work:
- Alternative computing architecture design
- Approximate Computing and error correction protocols
- Technical writing for research publications
Contact information for more details:
The commonly utilized Dynamic Random-access Memory (DRAM)- and Static Random-access Memory (SRAM)-based solutions lag in satisfying the memory requirements—capacity, latency, and energy—of modern applications and computing systems. The emerging Non-volatile Memory (NVM) technologies, such as Spin Transfer Torque Random Access Memory (STT-RAM), Phase-change Random-access Memory (PCRAM), Resistive Random-access Memory (ReRAM) and Racetrack Memory (RTM), offer a promising solution to overcome these bottlenecks. The NVMs offer better density and energy efficiency than the SRAM- and DRAM-based technologies. However, the NVMs have some limitations, such as variable access latency and costly write operations, which result in limited memory performance improvement. Furthermore, the NVM technologies are still in their exploratory phase compared to the SRAM technology. These challenges of NVMs open a research space for exploring various hardware and software architectures to overcome these challenges of NVMs and enable hybrid technologies-based memory hierarchies to improve memory systems’ performance.
In this work, we will focus on implementing an FPGA-based cycle-accurate emulator that can enable the quick analysis of various NVM-based caches on the overall performance of a computing system. The developed emulator would be evaluated for various benchmark applications.
Pre-requisites:
- Digital Design, Computer Architecture
- Knowledge of RISC-V architecture
- Experience with Xilinx Vivado, VHDL/Verilog
- Some scripting language (preferably Python), C++
Skills that will be acquired during project work:
- RISC-V-based System Design
- Knowledge about NVMs and caches
- System-level design and performance analysis
- FPGA Design tools
- Technical writing for research publications
Contact: Salim Ullah
Federated learning paradigm implies training on decentralized datasets. While several CPU/GPU-based servers can realize federated learning, a crucial requirement for IoT applications is to enable such a learning paradigm over decentralized hardware resources. The project requires exploring a hardware/software co-design approach towards federated learning. More information can be found in this Design and Test 2020 paper.
Pre-requisites: C/C++, Python, familiarity with git, Contact: Akash Kumar
The widespread use of electrical computing devices in many applications, including credit cards, smartphones, and autonomous cars, has significantly increased the importance of designing secure hardware. Hence, hardware manufacturers and designers spend a lot of time and money to ensure the security of their chips against various attacks. One of the common and effective attacks is side-channel attacks in which the attacker exploits unintended information leakage of the chip, such as power consumption, timing delay, electromagnetic radiation, etc., to extract secret information such as encryption key or circuit function. In recent years, emerging beyond CMOS technologies like spintronics and Reconfigurable Field-effect Transistors (RFETs) have provided unique abilities for securing hardware. This project aims to analyze the strengths and weaknesses of RFET circuits against traditional or ML-based side-channel attacks and propose efficient security solutions.
Pre-requisites:
- VLSI design knowledge
- SPICE and Cadence Virtuoso simulation
- Familiarity with HW security concepts
- Knowledge of Machine Learning (Favorable)
- Verilog
Contacts:
Nima Kavand (nima.kavand@tu-dresden.de), Armin Darjani (armin.darjani@tu-dresden.de)
As deep neural networks (DNNs) are evolving for solving complex real-world tasks, the need for computationally efficient algorithms is also growing to implement these networks in low powers devices. MobileNets, Binary Neural Networks, and Quantized DNNs are some examples of computationally efficient lightweight models. In recent years, studies demonstrate that deep learning systems can be fooled by carefully engineered adversarial examples with small imperceptible perturbations. There are many attacks and defense schemes proposed in this direction. This project aims to explore and implement methods to make lightweight neural networks robust against adversarial attacks.
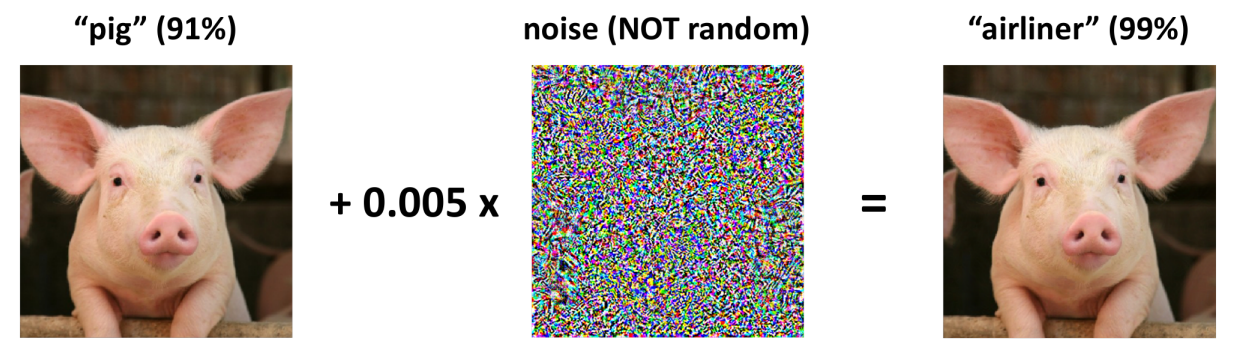
(Adversarial example https://gradientscience.org/intro_adversarial/)
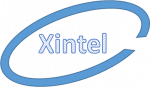
Most state-of-the-art FPGA-based arithmetic modules (in particular approximate modules) have considered Xilinx 6-input LUT structure. The availability of the 6-input LUTs and associated carry chains in Xilinx FPGAs enable resource-efficient and reduced latency designs. However, due to architectural differences, these designs cannot be directly imported to FPGAs from other vendors, e.g., Intel FPGAs (the second-highest FPGA market shareholder after Xilinx). The good thing is that Intel FPGAs also support 6-input fracturable LUTs and associated adders.
This project aims to develop a generic framework that will act as a bridge between Xilinx and Intel FPGAs. The framework will receive a design optimized for Xilinx FPGA and produce a corresponding design optimized for Intel FPGAs by utilizing the Intel FPGA primitives and vice versa. The obtained optimized design will be compared with other state-of-the-art designs and the Vendor-provided IPs. The framework will enable the easy adaptation of designs optimized for one FPGA vendor to another FPGA vendor.
Pre-requisites:
- Digital Design, Computer Architecture
- Xilinx FPGA architecture and design with VHDL/Verilog
- Experience with Xilinx Vivado
- Knowledge about Intel FPGAs (recommended)
- Knowledge about Intel Quartus (recommended)
- Some scripting language (preferably Python), C++
Skills that will be acquired during project work:
- FPGA Design tools (Xilinx and Intel)
- Designing with low-level primitives (LUTs and carry chains)
- Approximate Computing
- Technical writing for research publications
Related Publications:
- Ullah, Salim, Siva Satyendra Sahoo, Nemath Ahmed, Debabrata Chaudhury, and Akash Kumar. "AppAxO: Designing App lication-specific A ppro x imate O perators for FPGA-based Embedded Systems." ACM Transactions on Embedded Computing Systems (TECS) (2022).
- Ullah, Salim, Hendrik Schmidl, Siva Satyendra Sahoo, Semeen Rehman, and Akash Kumar. "Area-optimized accurate and approximate softcore signed multiplier architectures." IEEE Transactions on Computers 70, no. 3 (2020): 384-392.
Contact: Salim Ullah
In general, there are three categories of ML techniques -- supervised-learning, unsupervised-learning, and reinforcement-learning -- where depending on the problem, parameters, and inputs, only some of these techniques are suitable and used for system properties optimization. These ML techniques are memory-intensive and computationally expensive, which makes some of them incompatible with real-time system design due to the overheads, which may cause an effect on applications' timeliness. Therefore, this project aims to analyze and investigate various ML techniques in terms of overheads, accuracy, and capability and determine the efficient ones suitable for embedded real-time systems.
Pre-Requisites:
- Proficiency in C++, Python, Matlab
- Knowledge about Machine Learning techniques
- Good knowledge of computer architecture and algorithm design
Related Publications:
- S. Pagani, P. D. S. Manoj, A. Jantsch and J. Henkel, "Machine Learning for Power, Energy, and Thermal Management on Multicore Processors: A Survey," in IEEE Transactions on Computer-Aided Design of Integrated Circuits and Systems (TCAD), vol. 39, no. 1, pp. 101-116, 2020.
Contact: Behnaz Ranjbar (behnaz.ranjbar@tu-dresden.de)
A wide range of embedded systems found in the automotive and avionics industries are evolving into Mixed-Criticality (MC) systems to meet cost, space, timing, and power consumption requirements. In these MC systems, multiple tasks with different criticality levels are executed on common hardware. A failure occurring in tasks with different criticality levels has a disparate impact on the system, from no effect to catastrophic. These systems' functions, especially high-critical ones, must be ensured during their execution under various stresses (e.g., hardware errors, software errors, etc.) to prevent failure and catastrophic consequences. Therefore, to guarantee system safety, different reliability management techniques are employed to design such systems. In the case of fault occurrence, different techniques in different abstraction layers are needed to enhance their strengths against potential failures. Furthermore, due to the various safety demands for tasks, they can have different reliability requirements. This project aims to analyze the reliability management techniques across hardware and software layers of the system stack, which can be applied in MC applications, like automotive benchmarks.
Pre-Requisites:
- Proficiency in C++, Python, Matlab
- Good knowledge of computer architecture and algorithm design
- Strong architecture background with either general purpose multi-core platforms
Related Publications:
- Siva Satyendra Sahoo, Bharadwaj Veeravalli, Akash Kumar, "Cross-layer fault-tolerant design of real-time systems", In Proc. of International Symposium on Defect and Fault Tolerance in VLSI and Nanotechnology Systems (DFTS), pp. 1–6, 2016.
- Siva Satyendra Sahoo, Behnaz Ranjbar, Akash Kumar, "Reliability-Aware Resource Management in Multi-/Many-Core Systems: A Perspective Paper", In Journal of Low Power Electronics and Applications, MDPI AG, vol. 11, no. 1, pp. 7, 2021.
Contact: Behnaz Ranjbar (behnaz.ranjbar@tu-dresden.de)
A wide range of embedded systems found in the automotive and avionics industries are evolving into Mixed-Criticality (MC) systems, where multiple tasks with different criticality levels are executed on common hardware. In such MC systems, multiple Worst-Case Execution Times (WCETs) are defined for each task, corresponding to system operation mode to improve the MC system’s timing behavior at run-time. Determining different WCETs is one of the effective solutions in designing MC systems to improve confidence and safety, utilize resource usage, and execute more tasks on a platform. However, appropriate WCETs determination for lower criticality modes (low WCET) is non-trivial. Considering a very low WCET for tasks can improve the processor utilization by scheduling more tasks in that mode; on the other hand, using a larger WCET ensures that the mode switches are minimized, thereby improving the quality-of-service (QoS) for all tasks, albeit at the cost of processor utilization. However, determining the static low WCETs for tasks cannot adapt to dynamism at run-time. In this regard, in addition to MC system design at design-time, the run-time behavior of tasks needs to be considered by using Machine-Learning (ML) techniques that dynamically monitor the tasks’ execution times and adapt the low WCETs to environmental changes. This project aims to analyze the timing requirement of the MC systems at both design- and run-time by using ML techniques to improve the QoS and utilization. To achieve the project’s goal, several necessary hardware and software run-time controls may be used, and the MC applications are needed to be analyzed in terms of timing in all operation modes.
Pre-Requisites:
- Proficiency in C++, Python, Matlab
- Knowledge about Machine Learning techniques
- Good knowledge of computer architecture and algorithm design
- Strong architecture background with either general purpose multi-core platforms
Contact: Behnaz Ranjbar (behnaz.ranjbar@tu-dresden.de)
While Machine learning algorithms are being used for virtually every application, the high implementation costs of such algorithms still hinder their widespread use in resource-constrained Embedded systems. Approximate Computing (AxC) allows the designers to use low-energy (power and area) implementations with a slight degradation in results quality. Still better, Cross-layer Approximation (CLAx) offers the scope for much more improvements in power and energy reduction by using methods such as loop perforations, along with approximate hardware. Finding the proper combination of approximation techniques in hardware and software and across the layers of a DNN to provide just enough accuracy at the lowest cost poses an interesting research problem. In our research efforts towards solving this problem, we have implemented a DSE framework for 2D convolution. We would like to implement a similar framework for Convolution and Fully connected layers of a DNN.
- Pre-requisites:
- Digital Design, FPGA-based accelerator design, HLS
- Python, C++/ SystemC
- Skills that will be acquired during project-work:
- Hardware design for ML
- Multi-objective optimization of hardware accelerators.
- System-level design
- Technical writing for research publications.
- Related Publications:
- S. Ullah, S. S. Sahoo, A. Kumar, "CLAppED: A Design Framework for Implementing Cross-Layer Approximation in FPGA-based Embedded Systems" (to appear), In Proceeding: 2021 58th ACM/IEEE Design Automation Conference (DAC), pp. 1-6, Jul 2021.
- Ullah, H. Schmidl, S. S. Sahoo, S. Rehman, A. Kumar, "Area-optimized Accurate and Approximate Softcore Signed Multiplier Architectures", In IEEE Transactions on Computers, April 2020.
- Suresh Nambi, Salim Ullah, Aditya Lohana, Siva Satyendra Sahoo, Farhad Merchant, Akash Kumar, "ExPAN(N)D: Exploring Posits for Efficient Artificial Neural Network Design in FPGA-based Systems", 27 October 2020
- Contact: Salim Ullah
- Contact: Salim Ullah
- Contact: Salim Ullah