- Chair of Compiler Construction
- Chair of Emerging Electronic Technologies
- Chair of Knowledge-Based Systems
- Chair of Molecular Functional Materials
- Chair of Network Dynamics
- Chair of Organic Devices
- Chair of Processor Design
Network Dynamics - Challenges for our understanding
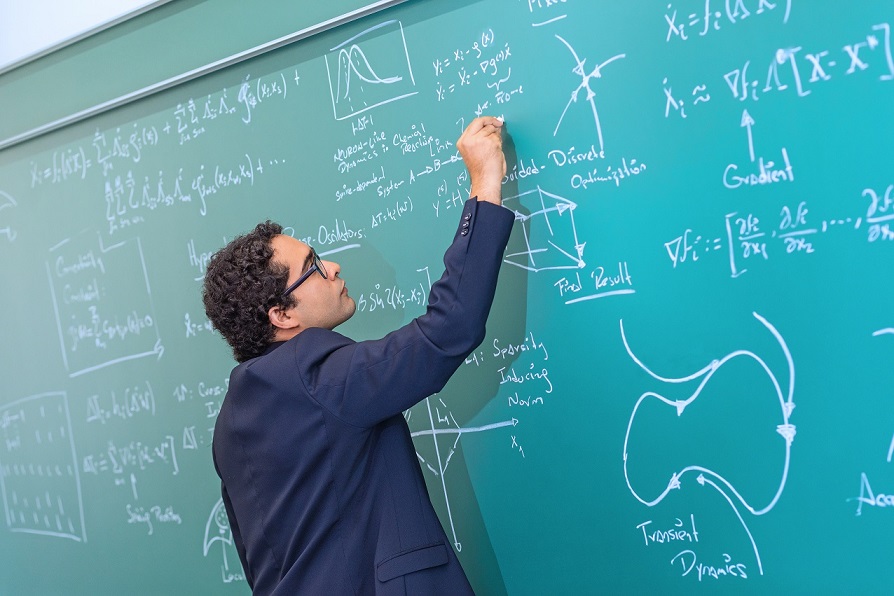
Networks are almost everywhere around us: We need the internet to display this text. We need neural networks in our brain to understand it. We need mobility networks to get us to the university and back home.
The goal of our research at the Chair for Network Dynamics is a unifying understanding of the fundamental mechanisms underlying the collective dynamics of all of these large, nonlinear, interconnected systems. We combine first-principles theory with data-driven analysis and modeling to investigate emergent phenomena in a broad range of network dynamical systems. A substantial part of our work focuses on developing conceptually new perspectives on complex systems and the theoretical and computational tools necessary to understand them.
This fundamental understanding forms the basis to predict and eventually control the dynamics of complex networked systems across disciplines.
Concepts and tools from network dynamics help us to understand a broad range of phenomena. We develop and transfer fundamental theoretical insights across applications towards a foundational understanding of network dynamical processes.
Methods developed to understand perturbation spreading in power grids or the emergence and structure of connectivity in general networked systems enable us to better understand the dynamics of epidemic spreading. Understanding response dynamics in networked systems allows us to solve complex inverse problems and infer the size of a system and the structure of its interactions from observations of few - or even a single - unit.
Humanity simultaneously faces multiple large and small-scale challenges affecting all aspects of society. Examples include the ongoing climate crisis and a simultaneous mass extinction of species, recurring financial crises, and currently a global pandemic. Addressing each of these challenges requires a deeper understanding of the emergent dynamics of complex systems. In addition, building blocks for solutions to one crisis often ignore or even compound the other problems. Successfully tackling all of these challenges requires an integrated approach combining concepts from multiple disciplines with a variety of theoretical methods and a broad range of empirical data and modeling tools. Understanding the complex interactions and emerging dynamics in these cross-disciplinary settings is at the heart of our complex systems research.
In the BMBF-funded project Krisenkomplex, we develop approaches to tackle such interacting challenges on the scientific, communication, and networking levels. We engage with experts in the individual fields to develop cross-disciplinary modelling approaches and predictions by building on an extensive array of conceptual, mathematical tools and complement these through advanced numerical modelling and data analysis. Moreover, we develop the initial foundations for a database to make these data sources and modelling approaches findable, accessible and reusable across disciplines by explicitly combining data management infrastructure with a simple documentation and understandable interface. In parallel, we collect and integrate best-practices of science communication and organization from complex systems' researchers around the world to understand workflows and organizational structures that help complex system science to tackle such challenges.
We illustrate these ideas by considering a current key challenge focusing on the interactions between two current problems, epidemic spreading and ecological sustainability in publicly accessible mobility: reducing the number of concurrent passengers in public transport reduces the risk of infection yet at the same time decreases the ecological sustainability. Building on the complex systems toolbox and the best-practice workflows, we answer how these opposing requirements can be reconciled.
KrisenKomplex - Revisiting resilience to crises with an integrated complex systems approach (BMBF project, 07/21 - 12/21)
idonate - Interacting Dynamics on Networks, Applications to Epidemiology (DFG Research Grant, 07/20 - 03/22)
Reinhart Koselleck-Project - Strongly Driven Nonlinear Network Dynamics (DFG, Reinhart Koselleck-Project, 01/25 - 12/29)
Collective Dynamics of Sustainable Mobility
Human mobility is changing rapidly, driven by digitalization and emerging technologies. A growing focus on sustainability reshapes mobility services and infrastructures everywhere. Battery-electric vehicles are replacing combustion engines. Digital technologies and autonomous vehicles promise to enable new forms of shared mobility.
In the research team Collective Dynamics of Sustainable Mobility, our objective is to reveal and understand the system-level collective dynamics emerging in our rapidly developing mobility landscape. We combine empirical data analysis and theoretical complex systems modeling with tools from statistical physics and nonlinear dynamics to investigate diverse topics. Which collective phenomena emerge in shared and networked mobility? How should we develop and design infrastructure and services to promote sustainable mobility? How can we integrate innovative sustainable mobility services with existing public transport?
Ultimately, we strive to provide mechanistic insights and analysis tools to contribute to the design and implementation of more effective, fair, and sustainable mobility solutions.
Find out more about our research below!
Ride-pooling extends ride-hailing services by combining multiple similar trips into the same vehicle. App-based ride-pooling services are a promising option for flexible, efficient, and more sustainable urban mobility. They theoretically offer the possibility to reduce both the number of cars on the road as well as the total vehicle kilometers traveled.
We study the collective dynamics of these services to understand the fundamental dynamics of the vehicle fleets and the structure of the routes of individual vehicles. Building on simplified numerical simulations and scaling arguments from statistical physics, we explain universal properties of the fleet dynamics and the efficiency of these services across a wide range of street networks. Based on these insights, we develop and test ideas to make these services more efficient and adaptive, for instance, by dynamically combing stops as well as trips.
Integrating multi-dimensional empirical data analysis and game-theoretical modeling approaches, we analyze the interactions, incentives, and decisions of the users, drivers, and service providers. For example, we describe and understand artificial price surges caused by the collective behavior of ride-hailing drivers and reveal fundamental mechanisms underlying a sharp transition towards acceptance of ride-pooling services.
Mobility infrastructure like bike paths or tram lines, flexible mobility options like on-demand ride-hailing services, and charging infrastructure for electric vehicles are critical to enabling sustainable mobility. However, in urban areas, insufficient infrastructure or flexibility may discourage cycling or the use of public transport. Similarly, sustainable mobility options are often lacking in rural areas, leaving private combustion engine cars as the only viable alternative.
We study the impact of the available infrastructure on the collective dynamics and the efficiency of sustainable mobility options. Modeling of long-range traffic of battery-electric vehicles reveals a new form of congestion emerging from drivers' attempts to avoid queues at charging stations. Building on insights into cyclists' route choices and algorithms from network science, we develop data-driven optimization approaches to suggest demand-aware bike path networks. Comparing the dynamics of ride-pooling services with the efficiency of traditional forms of public mobility, we study where on-demand mobility is feasible, under which conditions ride-pooling services become sustainable, and when line-based services are more efficient.
As the digitalization of mobility services continues, mobility as a whole becomes more networked. Digital routing apps are ubiquitous, computing the fastest routes on short- and long-range trips. Within a city, similar apps may suggest multi-modal trips combining different public transportation modes. New shared mobility services like bike-sharing or ride-pooling offer additional flexible mobility options.
While access to more information allows users to adjust to the current situation, the increased interactions between all participants may lead to unintended collective dynamics. We study under which conditions such detrimental effects emerge, how they influence the efficiency of different mobility services, and how to design services and systems to avoid these problems.
Using agent-based simulations and dynamical systems analysis, we investigate how access to routing information changes traffic flow and explain why it may cause congestion instead of preventing it. By developing abstract models capturing the defining features of different modes of transportation, we study the competition and the synergies arising from the fundamental collective interactions.
Where2Share - Ridepooling-Potential in Germany (BMDV mFund project, 07/23 - 12/24)
StaCyNet - Statistical physics of cycling infrastructure networks (DFG Research Grant, 06/22 - 05/25)
PrePara - Nonlinear dynamics of epidemic spreading in urban transport networks - impact of paratransit in a case study for Cape Town, South Africa (Volkswagen Foundation Initiative Corona Crisis and Beyond, 03/21 - 01/23)
Dynamics of Energy Systems, Climate and Sustainability
Energy fundamentally underlies all aspects of life. Its sustainable generation and reliable distribution are indispensable. We are currently transitioning from a fossil fuel-based energy system to one dominated by renewable sources. In addition, the same transition in other sectors, such as towards electric mobility, also affects the consumption of electricity. These drastic changes present an extraordinary challenge for the design and robust operation of future power grids and energy systems in general.
Our research focuses on principles of self-organization in high-dimensional dynamical systems to identify critical features of future-compliant energy systems. We build on fundamental aspects of dynamical systems theory, network dynamics, and time series analysis to characterize the dynamics of power grids and energy systems. This understanding enables us to predict and ultimately control instabilities emerging from the collective dynamics during the ongoing transition.
Our goal is to develop a fundamental understanding to support the integration of renewable energies and the transition to sustainable energy systems and usage.
Find out more about our research below!
Networked systems like power grids typically operate around a steady state. However, they are subject to various external perturbations. Changes in consumption patterns between day and night induce long-term oscillations in demand. Smaller-scale perturbations arise from power trading and control mechanisms. Finally, renewable sources or random outages may cause unpredictable fluctuations on arbitrary time scales.
We study the response dynamics of networked systems in fundamental theoretical models of linear and nonlinear response to reveal universal features of the dynamics across applications [1]. Together with data-driven analysis of the perturbations and fluctuations [2,3], we apply this understanding to power grids to model their response to fluctuating power input and demand.
With the transition to renewable sources, power generation relies on many smaller units and less reliable sources like wind or solar power. Changes in the power usage due to the transition to electric mobility mirror these changes on the consumption side. This spatially more distributed and temporally intermittent structure of generation and consumption provides significant challenges for the reliability and stability of future-compliant energy systems.
We analyze how the volatile, fluctuating, and distributed generation from renewable sources affects the operational stability of power grids. Building on fundamental mathematical modeling of the response dynamics in complex networks, we develop conceptual and computational tools to model and predict spatio-temporal response patterns as a function of the network structure and quantify the reliability of energy systems and individual units.
We apply these insights to answer questions like: Which fundamental principles underlie robust power generation and transmission networks? Which control mechanisms are most effective to prevent catastrophic failures? How can we economically extend an existing network to increase its robustness?
The transition to more sustainability influences all aspects of our daily lives, from electricity consumption over mobility to health and economic prosperity. Solutions for each individual aspect affect and often inhibit progress in other areas.
For example, a fast transition to electric mobility is desirable to reduce emissions. However, increased and more distributed electricity demand may enforce fossil fuel use and increase emissions. Moreover, the construction of electric vehicles and the required infrastructure may itself cause increased emissions and even completely counter the positive effects on time scales relevant to prevent significant global warming [1,2].
Integrating concepts from complex systems research and various application areas, we study the effects and interactions of different aspects to reveal and understand systemic, often counterintuitive effects. We aim to develop a fundamental understanding of the complex networked interactions to identify and demonstrate possibilities to simultaneously achieve multiple, seemingly conflicting goals towards a sustainable future.
SynCON - Synchronization and Collective Nonlinear Dynamics in Complexified Oscillator Networks (DFG Research Grant, 04/24 - 03/27)
ResiNet - Resilience of Power Grids against Perturbations (BMBF project, 03/24 - 12/24)
CoNDyNet2 - Collective Nonlinear Dynamics of Complex Power Grids (BMBF project, 01/19 - 04/22)
Network Dynamics of Natural Computation
Biological systems in general, and neuronal circuits in particular, exhibit collective dynamics that is self-organized, distributed, decentrally controlled, and often microscopically unreliable. Yet, they typically exhibit robust and predictable functions and can reliably solve a variety of computational tasks.
Spatially and temporally coordinated patterns of neural activity are key to information processing in the brain. Yet, their dynamical origin and, more generally, the mechanisms underlying how distributed activity yields nonlinear computations in the brain are far from fully understood.
We study how heterogeneous interaction networks, non-additive coupling, and other nonlinearities act together to induce specific coordinated activity patterns and how neural and bio-inspired dynamical systems coordinate activity and thus effectively process, route, and transmit information.
TransparNet - Predictable collective dynamics of bio-inspired reservoir networks - towards transparent neural network computing (Sächsische Aufbaubank (SAB) Forschungsprojektförderung TG 70, 01/21 - 09/22)
Heterokline Netzwerke - From nonlinear dynamics to proof of concept for Heteroclinic Computing (DFG Research Grant, 07/19 - 08/22)